mashr analysis after dreamlet
Borrowing information across genes and cell types
Developed by Gabriel Hoffman
Run on 2023-08-09 09:18:56.878897
Source:vignettes/mashr.Rmd
mashr.Rmd
Instroduction
mashr
is a Bayesian statistical method to borrow information across genes and
cell type (Urbut,
et al, 2019). mashr
takes estimated log fold changes and standard errors for each cell type
and gene from dreamlet
, and produces posterior estimates
with more accuracy and precision then the original parameter
estimates.
Standard dreamlet
analysis
Preprocess data
Here single cell RNA-seq data is downloaded from ExperimentHub
library(dreamlet)
library(muscat)
library(ExperimentHub)
library(zenith)
library(scater)
# Download data, specifying EH2259 for the Kang, et al study
eh <- ExperimentHub()
sce <- eh[["EH2259"]]
# only keep singlet cells with sufficient reads
sce <- sce[rowSums(counts(sce) > 0) > 0, ]
sce <- sce[,colData(sce)$multiplets == 'singlet']
# compute QC metrics
qc <- perCellQCMetrics(sce)
# remove cells with few or many detected genes
ol <- isOutlier(metric = qc$detected, nmads = 2, log = TRUE)
sce <- sce[, !ol]
# compute normalized data
sce <- sce[rowSums(counts(sce) > 1) >= 10, ]
sce <- computeLibraryFactors(sce)
sce <- logNormCounts(sce)
# set variable indicating stimulated (stim) or control (ctrl)
sce$StimStatus = sce$stim
Aggregate to pseudobulk
# Since 'ind' is the individual and 'StimStatus' is the stimulus status,
# create unique identifier for each sample
sce$id <- paste0(sce$StimStatus, sce$ind)
# Create pseudobulk data by specifying cluster_id and sample_id
# Count data for each cell type is then stored in the `assay` field
# assay: entry in assayNames(sce) storing raw counts
# cluster_id: variable in colData(sce) indicating cell clusters
# sample_id: variable in colData(sce) indicating sample id for aggregating cells
pb <- aggregateToPseudoBulk(sce,
assay = "counts",
cluster_id = "cell",
sample_id = "id",
verbose = FALSE)
dreamlet
for pseudobulk
# Normalize and apply voom/voomWithDreamWeights
res.proc = processAssays( pb, ~ StimStatus, min.count=5)
# Differential expression analysis within each assay,
# evaluated on the voom normalized data
res.dl = dreamlet( res.proc, ~ StimStatus)
Run mashr
analysis
# run mashr model to borrow information across genes and
# cell types in estimating coefficients' posterior distribution
res_mash = run_mash(res.dl, coef='StimStatusstim')
Summarize mashr
results
Compute summary of mashr posterior distributions
library(mashr)
# extract statistics from mashr model
# NA values indicate genes not sufficiently expressed
# in a given cell type
# original logFC
head(res_mash$logFC.original)[1:4, 1:4]
## B cells CD14+ Monocytes CD4 T cells CD8 T cells
## A1BG NA NA -0.73718671 NA
## AAAS NA NA -0.56991157 NA
## AAED1 NA 1.426001 0.07140051 NA
## AAK1 NA NA -0.91972740 NA
## B cells CD14+ Monocytes CD4 T cells CD8 T cells
## A1BG NA NA -0.6327307 NA
## AAAS NA NA -0.4543872 NA
## AAED1 NA 1.378843 0.0201326 NA
## AAK1 NA NA -0.8578750 NA
# how many gene-by-celltype tests are significant
# i.e. if a gene is significant in 2 celltypes, it is counted twice
table(get_lfsr(res_mash$model) < 0.05, useNA="ifany")
##
## FALSE TRUE <NA>
## 8089 6073 30134
# how many genes are significant in at least one cell type
table( apply(get_lfsr(res_mash$model), 1, min, na.rm=TRUE) < 0.05)
##
## FALSE TRUE
## 2568 2969
# how many genes are significant in each cell type
apply(get_lfsr(res_mash$model), 2, function(x) sum(x < 0.05, na.rm=TRUE))
## B cells CD14+ Monocytes CD4 T cells CD8 T cells
## 767 2086 1525 412
## Dendritic cells FCGR3A+ Monocytes Megakaryocytes NK cells
## 52 566 36 629
# examine top set of genes
# which genes are significant in at least 1 cell type
sort(names(get_significant_results(res_mash$model)))[1:10]
## [1] "ACTB" "ACTG1_ENSG00000184009" "ARPC1B"
## [4] "ATP6V0E1" "B2M" "BTF3"
## [7] "BTG1" "CALM2" "CD74"
## [10] "CFL1"
# There is a lot of variation in the raw logFC
res_mash$logFC.original["ISG20",]
## B cells CD14+ Monocytes CD4 T cells CD8 T cells
## 3.200534 5.865638 3.060855 3.533391
## Dendritic cells FCGR3A+ Monocytes Megakaryocytes NK cells
## 3.593594 4.370017 NA 3.577744
# posterior mean after borrowing across cell type and genes
get_pm(res_mash$model)["ISG20",]
## B cells CD14+ Monocytes CD4 T cells CD8 T cells
## 3.201633 5.807546 3.063965 3.535864
## Dendritic cells FCGR3A+ Monocytes Megakaryocytes NK cells
## 3.601904 4.350143 NA 3.577692
Gene set analysis
Perform gene set analysis with zenith
using posterior
mean for each coefficient
# gene set analysis using mashr results
library(zenith)
# Load Gene Ontology database
# use gene 'SYMBOL', or 'ENSEMBL' id
# use get_MSigDB() to load MSigDB
go.gs = get_GeneOntology("CC", to="SYMBOL")
# valid values for statistic:
# "tstatistic", "abs(tstatistic)", "logFC", "abs(logFC)"
df_gs = zenith_gsa(res_mash, go.gs)
# Heatmap of results
plotZenithResults(df_gs, 5, 1)
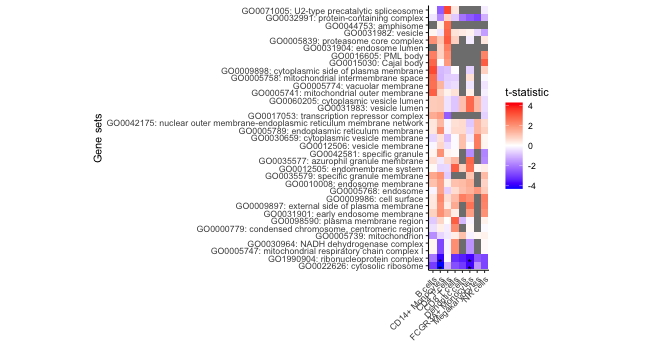
plot of chunk zenith
# forest plot based on mashr results
plotForest(res_mash, "ISG20")
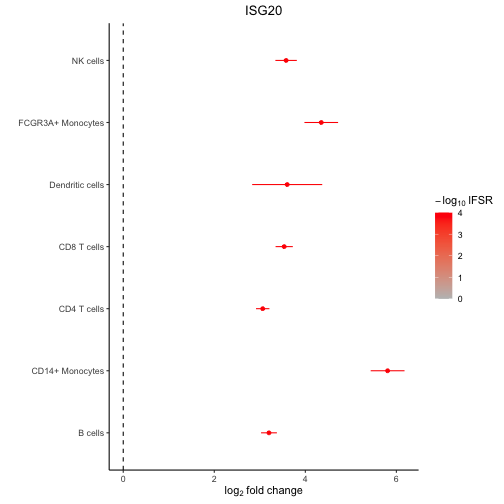
plot of chunk forest
Volcano plot based on local False Sign Rate (lFSR) estimated from the posterior distribution of each coefficient.
# volcano plot based on mashr results
# yaxis uses local false sign rate (lfsr)
plotVolcano(res_mash)
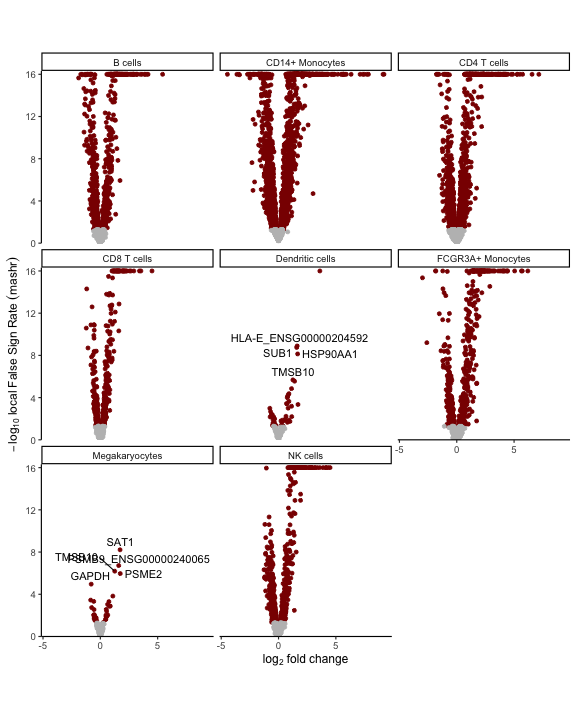
plot of chunk volcano
Session Info
## R version 4.3.0 (2023-04-21)
## Platform: x86_64-apple-darwin22.4.0 (64-bit)
## Running under: macOS Ventura 13.5
##
## Matrix products: default
## BLAS: /Users/gabrielhoffman/prog/R-4.3.0/lib/libRblas.dylib
## LAPACK: /usr/local/Cellar/r/4.3.0_1/lib/R/lib/libRlapack.dylib; LAPACK version 3.11.0
##
## locale:
## [1] en_US.UTF-8/en_US.UTF-8/en_US.UTF-8/C/en_US.UTF-8/en_US.UTF-8
##
## time zone: America/New_York
## tzcode source: internal
##
## attached base packages:
## [1] stats4 stats graphics grDevices datasets utils methods
## [8] base
##
## other attached packages:
## [1] mashr_0.2.69 ashr_2.2-54
## [3] muscData_1.14.0 scater_1.28.0
## [5] scuttle_1.10.1 SingleCellExperiment_1.22.0
## [7] SummarizedExperiment_1.30.1 Biobase_2.60.0
## [9] GenomicRanges_1.52.0 GenomeInfoDb_1.36.1
## [11] IRanges_2.34.1 S4Vectors_0.38.1
## [13] MatrixGenerics_1.12.0 matrixStats_1.0.0
## [15] zenith_1.3.0 ExperimentHub_2.8.0
## [17] AnnotationHub_3.8.0 BiocFileCache_2.8.0
## [19] dbplyr_2.3.2 BiocGenerics_0.46.0
## [21] muscat_1.14.0 dreamlet_0.99.23
## [23] variancePartition_1.31.11 BiocParallel_1.34.2
## [25] limma_3.56.2 ggplot2_3.4.2
##
## loaded via a namespace (and not attached):
## [1] bitops_1.0-7 httr_1.4.6
## [3] RColorBrewer_1.1-3 doParallel_1.0.17
## [5] Rgraphviz_2.44.0 numDeriv_2016.8-1.1
## [7] tools_4.3.0 sctransform_0.3.5
## [9] backports_1.4.1 utf8_1.2.3
## [11] R6_2.5.1 GetoptLong_1.0.5
## [13] withr_2.5.0 prettyunits_1.1.1
## [15] gridExtra_2.3 cli_3.6.1
## [17] labeling_0.4.2 KEGGgraph_1.60.0
## [19] SQUAREM_2021.1 mvtnorm_1.2-2
## [21] blme_1.0-5 mixsqp_0.3-48
## [23] parallelly_1.36.0 invgamma_1.1
## [25] RSQLite_2.3.1 generics_0.1.3
## [27] shape_1.4.6 gtools_3.9.4
## [29] dplyr_1.1.2 Matrix_1.5-4.1
## [31] ggbeeswarm_0.7.2 fansi_1.0.4
## [33] abind_1.4-5 lifecycle_1.0.3
## [35] yaml_2.3.7 edgeR_3.42.4
## [37] gplots_3.1.3 grid_4.3.0
## [39] blob_1.2.4 promises_1.2.0.1
## [41] crayon_1.5.2 lattice_0.21-8
## [43] beachmat_2.16.0 msigdbr_7.5.1
## [45] annotate_1.78.0 KEGGREST_1.40.0
## [47] pillar_1.9.0 knitr_1.43
## [49] ComplexHeatmap_2.16.0 rjson_0.2.21
## [51] boot_1.3-28.1 corpcor_1.6.10
## [53] future.apply_1.11.0 codetools_0.2-19
## [55] glue_1.6.2 data.table_1.14.8
## [57] vctrs_0.6.3 png_0.1-8
## [59] Rdpack_2.4 gtable_0.3.3
## [61] assertthat_0.2.1 cachem_1.0.8
## [63] xfun_0.39 rbibutils_2.2.13
## [65] S4Arrays_1.0.4 mime_0.12
## [67] Rfast_2.0.7 iterators_1.0.14
## [69] interactiveDisplayBase_1.38.0 ellipsis_0.3.2
## [71] nlme_3.1-162 pbkrtest_0.5.2
## [73] bit64_4.0.5 progress_1.2.2
## [75] EnvStats_2.7.0 filelock_1.0.2
## [77] TMB_1.9.4 irlba_2.3.5.1
## [79] vipor_0.4.5 KernSmooth_2.23-21
## [81] colorspace_2.1-0 rmeta_3.0
## [83] DBI_1.1.3 DESeq2_1.40.1
## [85] tidyselect_1.2.0 bit_4.0.5
## [87] compiler_4.3.0 curl_5.0.0
## [89] graph_1.78.0 BiocNeighbors_1.18.0
## [91] DelayedArray_0.26.3 scales_1.2.1
## [93] caTools_1.18.2 remaCor_0.0.17
## [95] rappdirs_0.3.3 stringr_1.5.0
## [97] digest_0.6.33 minqa_1.2.5
## [99] aod_1.3.2 XVector_0.40.0
## [101] RhpcBLASctl_0.23-42 htmltools_0.5.5
## [103] pkgconfig_2.0.3 lme4_1.1-33
## [105] sparseMatrixStats_1.12.0 highr_0.10
## [107] fastmap_1.1.1 rlang_1.1.1
## [109] GlobalOptions_0.1.2 shiny_1.7.4
## [111] DelayedMatrixStats_1.22.0 farver_2.1.1
## [113] BiocSingular_1.16.0 RCurl_1.98-1.12
## [115] magrittr_2.0.3 GenomeInfoDbData_1.2.10
## [117] munsell_0.5.0 Rcpp_1.0.11
## [119] babelgene_22.9 viridis_0.6.3
## [121] EnrichmentBrowser_2.30.1 RcppZiggurat_0.1.6
## [123] stringi_1.7.12 zlibbioc_1.46.0
## [125] MASS_7.3-60 plyr_1.8.8
## [127] parallel_4.3.0 listenv_0.9.0
## [129] ggrepel_0.9.3 Biostrings_2.68.1
## [131] splines_4.3.0 hms_1.1.3
## [133] circlize_0.4.15 locfit_1.5-9.7
## [135] reshape2_1.4.4 ScaledMatrix_1.8.1
## [137] BiocVersion_3.17.1 XML_3.99-0.14
## [139] evaluate_0.21 BiocManager_1.30.20
## [141] nloptr_2.0.3 foreach_1.5.2
## [143] httpuv_1.6.11 tidyr_1.3.0
## [145] purrr_1.0.1 future_1.32.0
## [147] clue_0.3-64 scattermore_1.1
## [149] rsvd_1.0.5 broom_1.0.5
## [151] xtable_1.8-4 fANCOVA_0.6-1
## [153] later_1.3.1 viridisLite_0.4.2
## [155] truncnorm_1.0-9 tibble_3.2.1
## [157] lmerTest_3.1-3 glmmTMB_1.1.7
## [159] memoise_2.0.1 beeswarm_0.4.0
## [161] AnnotationDbi_1.62.1 cluster_2.1.4
## [163] globals_0.16.2 GSEABase_1.62.0